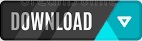
However, since many subjects are studied by different groups of students, they can only be clustered on the basis of soft clustering. Now, in order to do so, the administration department will have to identify common classes of 20 students in a batch. In order to cluster students on the basis of the subjects they study, the administration will have to figure out the maximum similarity between these students. Herein, we shall consider a group of high-school students in the West as a group of data points in a given dataset. Let us understand the types of cluster analysis with the help of a common cluster analysis example. (Related blog: A Fuzzy-Logic Approach In Decision-Making) This means that a fuzzy classification of datasets characterizes soft clustering.įuzzy Clustering Algorithm in Machine learning is a renowned unsupervised algorithm for processing data into soft clusters. In the case of soft clustering, a given data point can belong to more than one cluster at a time.

Unlike hard clustering that requires a given data point to belong to only a cluster at a time, soft clustering follows a different rule. The second class of cluster analysis is Soft Clustering. However, there are no other grounds of similarity with data sets belonging to other clusters. This implies that a hard-core classification of datasets is required in order to organize and classify data accordingly.įor instance, a clustering algorithm classifies data points in one cluster such that they have the maximum similarity. In a given dataset, it is possible for a data researcher to organize clusters in a manner that a single dataset is placed in only one of the total number of given clusters. On the basis of the categorization of data sets into a particular cluster, cluster analysis can be divided into 2 types - hard and soft clustering. "Homogeneity plays a crucial role in clustering as the algorithms learn to identify similar grounds in datasets that are provided to the machines."īroadly, there are 2 types of cluster analysis methods. They are as follows - centroid-based, density-based, distribution-based, hierarchical, constraint-based, and fuzzy clustering. When it comes to Machine learning algorithms, clustering in machine learning is a prominent statistical technique that has evolved over time.Ī set of clustering algorithms, empowered by the duo of cluster analysis and machine learning, have emerged on the surface that enables organizations and industries to run through their data and cluster data in an organized manner.Ĭlustering is one of the most renowned unsupervised machine learning algorithms that has been known to humankind.īroadly, there are 6 types of clustering algorithms in Machine learning. Cluster analysis is an inductive exploratory technique in the sense that it uncovers structures without explaining the reasons for their existence." "Researchers are often confronted with the task of sorting observed data into meaningful structures. With the help of a set of algorithms empowered by Machine learning, cluster analysis discriminates among various data points in such a way that those with maximum differences are placed in different clusters. It must be noted that the level of similarity between two data points in one cluster is maximum while the similarity between two data points of different clusters is minimum. Rightly put, cluster analysis is a way of putting data points with similar characteristics in one group so that they differ from other data points of other clusters.

Among a variety of techniques, cluster analysis is one such statistical method.Ĭluster analysis in statistics is a method to organize data by clustering data points in a particular cluster.

Furthermore, data analytics has become a major operation in the field of data science as it helps to derive patterns from vast amounts of data for making future decisions for the betterment of an organization.Ī number of techniques under this concept are incorporated to organize data and make it more approachable. That said, statistics help a lot in achieving this purpose. With loads of data flooding organizations, it is important to organize it and keep substantial records of it. Data science is a vast field that is operational in almost every industrial sector today.
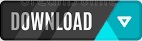